# Example Code for the Optimum Filters¶
Import qetpy
and other necessary packages.
import numpy as np
import matplotlib.pyplot as plt
import qetpy as qp
from pprint import pprint
Use QETpy
to generate some simulated TES noise¶
We can use qetpy.sim.TESnoise
to help create a simulated PSD with
characteristic TES parameters.
fs = 625e3
f = np.fft.fftfreq(32768, d=1/fs)
noisesim = qp.sim.TESnoise(r0=0.03)
psd_sim = noisesim.s_iload(freqs=f) + noisesim.s_ites(freqs=f) + noisesim.s_itfn(freqs=f)
f_fold, psd_sim_fold = qp.foldpsd(psd_sim, fs=fs)
fig, ax = plt.subplots(figsize=(8, 6))
ax.loglog(f_fold, psd_sim_fold**0.5, color="blue", alpha=0.5, label="Simulated PSD, No Spikes")
ax.set_ylim(1e-12,1e-9)
ax.grid()
ax.grid(which="minor", linestyle="dotted")
ax.tick_params(which="both", direction="in", right=True, top=True)
ax.legend(loc="best")
ax.set_title("Simulated Current PSD")
ax.set_ylabel("PSD [A/$\sqrt{\mathrm{Hz}}$]")
ax.set_xlabel("Frequency [Hz]")
fig.tight_layout()
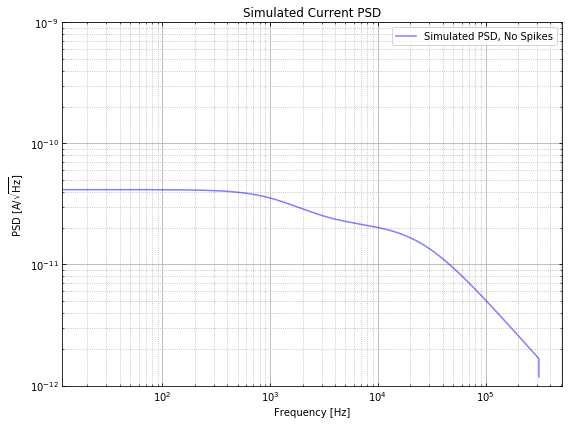
With a PSD, we can use qetpy.gen_noise
to generate random noise from
the PSD (assuming the frequencies are uncorrelated). Then, we will
create an example pulse.
# create a template
pulse_amp = 1e-6 # [A]
tau_f = 66e-6 # [s]
tau_r = 20e-6 # [s]
t = np.arange(len(psd_sim))/fs
pulse = np.exp(-t/tau_f)-np.exp(-t/tau_r)
pulse_shifted = np.roll(pulse, len(t)//2)
template = pulse_shifted/pulse_shifted.max()
# use the PSD to create an example trace to fit
noise = qp.gen_noise(psd_sim, fs=fs, ntraces=1)[0]
signal = noise + np.roll(template, 100)*pulse_amp # note the shift we have added, 160 us
Fit a single pulse with OptimumFilter
¶
With a pulse created, let’s use the qetpy.OptimumFilter
class to run
different Optimum Filters.
qp.OptimumFilter?
Below, we print the different methods available in
qetpy.OptimumFilter
. In this notebook, we will demo the
ofamp_nodelay
, ofamp_withdelay
, ofamp_pileup_iterative
, and
update_signal
methods. It is highly recommend to read the
documentation for the other methods, as there are many useful ones!
method_list = sorted([func for func in dir(qp.OptimumFilter) if callable(getattr(qp.OptimumFilter, func)) and not func.startswith("__")])
pprint(method_list)
['chi2_lowfreq',
'chi2_nopulse',
'energy_resolution',
'ofamp_baseline',
'ofamp_nodelay',
'ofamp_pileup_iterative',
'ofamp_pileup_stationary',
'ofamp_withdelay',
'time_resolution',
'update_signal']
Let’s run the Optimum Filter without and with a time-shifting degree of freedom.
OF = qp.OptimumFilter(signal, template, psd_sim, fs) # initialize the OptimumFilter class
amp_nodelay, chi2_nodelay = OF.ofamp_nodelay()
amp_withdelay, t0_withdelay, chi2_withdelay = OF.ofamp_withdelay()
print(f"No Delay Fit: amp = {amp_nodelay*1e6:.2f} μA, χ^2 = {chi2_nodelay:.2f}")
print(f"With Delay Fit: amp = {amp_withdelay*1e6:.2f} μA, t_0 = {t0_withdelay*1e6} μs, χ^2 = {chi2_withdelay:.2f}")
No Delay Fit: amp = -0.04 μA, χ^2 = 210399.75
With Delay Fit: amp = 1.00 μA, t_0 = 160.0 μs, χ^2 = 32407.30
Since we have added a 160 us shift, we see that the “with delay” optimum filter fit the time-shift perfectly, and the chi-squared is very close to the number of degrees of freedom (32768), as we would expect for a good fit.
fig, ax = plt.subplots(figsize=(10, 6))
ax.plot(t*1e6, signal*1e6, label="Simulated Pulse", color="blue", alpha=0.5)
ax.plot(t*1e6, amp_withdelay*np.roll(template, int(t0_withdelay*fs))*1e6,
label="Fit With Delay", color="red", linestyle="dotted")
ax.set_ylabel("Current [μA]")
ax.set_xlabel("Time [μs]")
ax.set_title("Simulated Data")
lgd = ax.legend(loc="upper left")
ax.tick_params(which="both", direction="in", right=True, top=True)
ax.grid(linestyle="dotted")
fig.tight_layout()
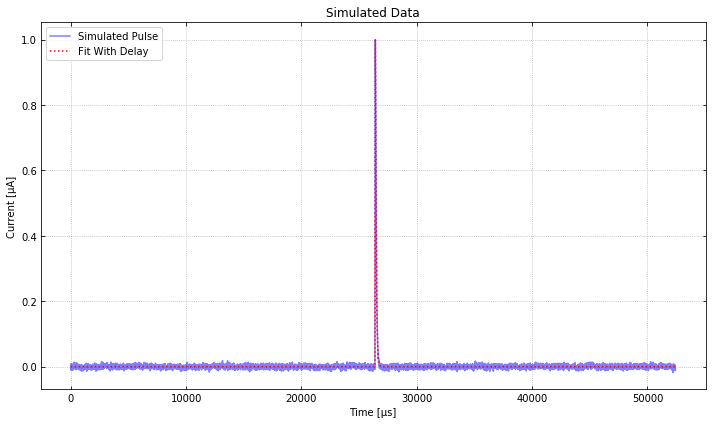
Add a pileup pulse and fit with OptimumFilter
¶
Let’s now add a second (pileup) pulse in order to see how we can use
ofamp_pileup_iterative
.
pileup = signal + np.roll(template, 10000)*pulse_amp
OF.update_signal(pileup) # update the signal in order to fit a new trace
amp_withdelay, t0_withdelay, chi2_withdelay = OF.ofamp_withdelay(nconstrain=300)
amp_pileup, t0_pileup, chi2_pileup = OF.ofamp_pileup_iterative(amp_withdelay, t0_withdelay)
print(f"With Delay Fit: amp = {amp_withdelay*1e6:.2f} μA, t_0 = {t0_withdelay*1e6} μs, χ^2 = {chi2_withdelay:.2f}")
print(f"Pileup Fit: amp = {amp_pileup*1e6:.2f} μA, t_0 = {t0_pileup*1e6} μs, χ^2 = {chi2_pileup:.2f}")
With Delay Fit: amp = 1.00 μA, t_0 = 160.0 μs, χ^2 = 209503.04
Pileup Fit: amp = 1.00 μA, t_0 = 16000.0 μs, χ^2 = 32407.28
As expected, the pileup optimum filter fit the data very well, as we can see from the chi-squared above.
fig, ax = plt.subplots(figsize=(10, 6))
ax.plot(t*1e6, pileup*1e6, label="Simulated Pileup Pulse", color="blue", alpha=0.5)
ax.plot(t*1e6, amp_withdelay*np.roll(template, int(t0_withdelay*fs))*1e6 + \
amp_pileup*np.roll(template, int(t0_pileup*fs))*1e6,
label="Pileup Pulse Fit", color="red", linestyle="dotted")
ax.set_ylabel("Current [μA]")
ax.set_xlabel("Time [μs]")
ax.set_title("Simulated Data")
lgd = ax.legend(loc="upper left")
ax.tick_params(which="both", direction="in", right=True, top=True)
ax.grid(linestyle="dotted")
fig.tight_layout()
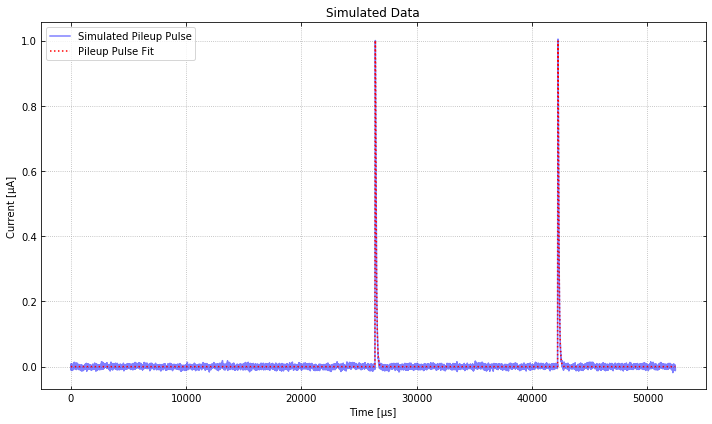
Nonlinear Fitting¶
What about when we do not have a template? The qetpy.OFnonlin
class
has been written to fit the fall times as well, which is useful for
cases where we do not have a template, or we know that the template will
not match the data well.
pulse_amp = 1e-6
tau_f = 160e-6
tau_r = 20e-6
t = np.arange(len(psd_sim))/fs
pulse = np.exp(-t/tau_f)-np.exp(-t/tau_r)
pulse_shifted = np.roll(pulse, len(t)//2)
template = pulse_shifted/pulse_shifted.max()
noise = qp.gen_noise(psd_sim, fs=fs, ntraces=1)[0]
signal = noise + np.roll(template, 100)*pulse_amp
We can try using our “bad” template (with a 66 us fall time), but we will see that the chi-squared indicates a non-ideal fit.
OF.update_signal(signal) # update the signal in order to fit a new trace
amp_withdelay, t0_withdelay, chi2_withdelay = OF.ofamp_withdelay(nconstrain=300)
print(f"With Delay Fit: amp = {amp_withdelay*1e6:.2f} μA, t_0 = {t0_withdelay*1e6:.2f} μs, χ^2 = {chi2_withdelay:.2f}")
With Delay Fit: amp = 1.07 μA, t_0 = 163.20 μs, χ^2 = 72524.20
Let’s use qetpy.OFnonlin
to do the fit. To help visualize the fit,
we will use the parameter lgcplot=True
to plot the fit in frequency
domain and time domain
qp.OFnonlin?
qp.OFnonlin.fit_falltimes?
nonlinof = qp.OFnonlin(psd_sim, fs, template=None)
params, error, _, chi2_nonlin, success = nonlinof.fit_falltimes(signal, npolefit=2, lgcfullrtn=True, lgcplot=True)
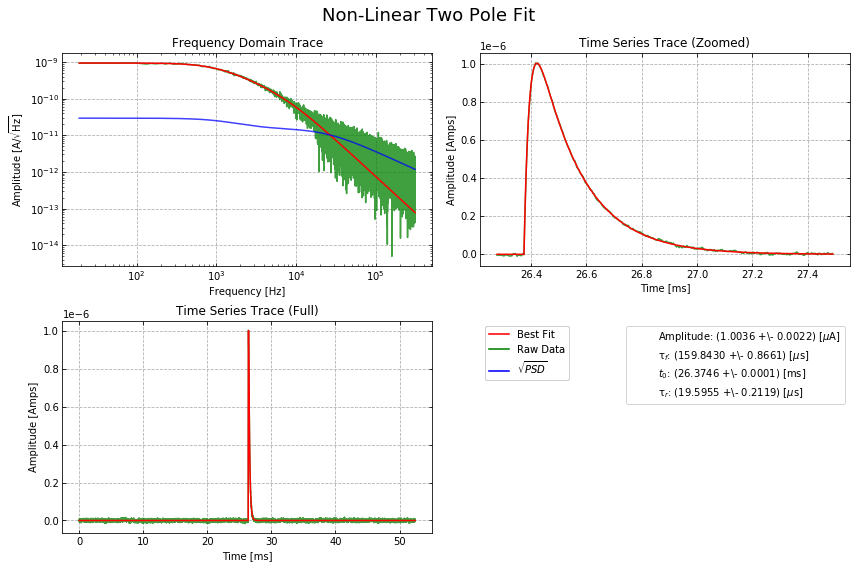
print(f"Nonlinear fit: χ^2 = {chi2_nonlin * (len(nonlinof.data)-nonlinof.dof):.2f}")
Nonlinear fit: χ^2 = 32719.95
And we see that the fit using qetpy.OFnonlin
is great! The
chi-squared is near the number of degrees of freedom (32768), which
indicates that we have a good fit.
For further documentation on the different fitting functions, please visit https://qetpy.readthedocs.io/en/latest/qetpy.core.html#module-qetpy.core._fitting.